Several times I have written about the need for AI legislation, especially since the AI landscape sometimes seems like the wild west. Canada’s bill C-27 is one of the responses from the government that I expected.
In this article, I’ll explain what C-27 is, how your company will be impacted by it, and my take on its impact on the artificial intelligence field.
What is C-27?
C-27 is the Act to enact the Consumer Privacy Protection Act, the Personal Information and Data Protection Tribunal Act and the Artificial Intelligence and Data Act and to make consequential and related amendments to other Acts.
Wow, what a long-winded name! Essentially, C-27, also called the “Digital Charter Implementation Act”, is a Canadian law proposal that was released in June 2022 with the aim to protect personal data about individuals. C-27 could be considered the equivalent of a “modernized” GDPR (General Data Protection Regulation) in Europe, with a broader scope, given that it covers AI systems’ trustworthiness and privacy rights.
This Act is comprehensive and applies to personal information that organizations collect, use, or disclose in the course of commercial activities; or personal information about an employee of, or an applicant for employment with, the organization and that the organization collects, uses, or discloses in connection with the operation of a federal work, undertaking, or business.
What C-27 means in plain English
Essentially, this act ensures that companies take the privacy of their customers’ and employees’ information they collect, use, or disclose seriously.
What are the key differences between GDPR and C-27?
Although they use different clauses and terms, C-27 basically covers the same rights as GDPR (rights to access, opt-out of direct marketing, data portability, or erasure). However, the scope of C-27 is broader, as it explicitly covers employee data.
C-27 also explicitly covers artificial intelligence applications since they use and generate data. More specifically, this Act will require that:
- Consumers or employees impacted by an AI application can request clear and specific explanations related to the system prediction.
- High-impact AI applications perform an evaluation of potential negative biases and unjustified discrimination that may negatively treat specific populations or individuals.
- High-impact AI applications document risks associated with negative biases or harmful outcomes of the application, identify mitigation strategies, and demonstrate the monitoring of these risks.
Why should you care about Canada’s bill C-27?
First, it is a necessary legislative document to ensure that data about Canadian residents is kept secure. For example, only six months after the slow and painful implementation of GDPR in Europe, 44% of respondents in a Deloitte poll believe that organizations care more about their customers’ privacy now that GDPR is in force. This is powerful.
However, this means that a considerable amount of work must be undertaken to comply with C-27. Almost half of all European organizations have made a significant investment in their GDPR compliance capabilities, and 70% of organizations have seen an increase in staff that are partly or entirely focused on GDPR compliance. However, 45% of these organizations are still not compliant to GDPR. According to the GDPR enforcement tracker, since July 2018, 1317 fines have been issued.
Is C-27 going to generate as much chaos for Canadian companies? Probably not. Canadian organizations have already started to adapt to this new era of data privacy. GDPR is not new anymore; it was announced in 2016 and took effect in May 2018. We have learned a lot since then. For example, 85% of Canadian organizations have already appointed a Chief Data Protection Officer (CDPO), and most third-party tools have adapted their products and services to respect data privacy.
In other words:
- C-27 is going to be implemented. This is certain.
- This is serious. In Europe, about 20% of individuals have already used their rights through the GDPR.
- The more proactive you are, the more straightforward and painless your implementation will be.
- It is not the end of the world. You can be compliant without spending millions of dollars.
All that said, you must start preparing your organization for the implementation of C-27.
Here are four actions you can take right now to be prepared for C-27
1. Control your data collection and management processes.
Maintain good data hygiene so that you will be able to better control personal data in your different tools, systems, and databases.
2. Start embracing data de-identification techniques to minimize the footprint of personal information in your organization.
A great way to limit the amount of personal data flowing into your databases is by limiting its usage. This can be done by eliminating or reducing the number of databases, tables, and fields containing personal data, which will significantly reduce the complexity of complying to C-27. Here are a few de-identification techniques:
- De-identify: modify personal information to reduce the chances that an individual can be directly identified from it.
Hashing methods are an example of de-identification as business users cannot identify individuals using the data. Still, the IT and Security teams can convert the hashes into identifiable data if required. De-identification techniques are allowed if appropriate processes and policies are in place to safeguard them.
In AI systems, de-identification techniques still allow for predictive power. For example, without knowing an exact zip code, individuals from zip code 12345 will have similar characteristics. However, their predictive power is limited compared to the actual data. For example, it is impossible to calculate the distance between zip codes if they are hashed. - Anonymize: modify personal information irreversibly and permanently in accordance with generally accepted best practices to ensure that no individual can be identified from the information, whether directly or indirectly, by any means.
This is a rigorous privacy method that should not be the default in a data science strategy. By default, organizations should de-identify the data as much as they can and only use anonymization when there is no other choice. For example, free form texts and call transcriptions can contain very private and identifiable information that is quite complex to de-identify. In those cases, anonymization is required. - Generate synthetic data: create completely fake and realistic data based on existing data so that it is possible to develop analytics and AI applications without risking privacy issues.
Nowadays, many tools and algorithms let organizations generate realistic synthetic data without jeopardizing real personal data. This technique enables organizations to build AI applications with any type of data, identifiable or not, on tabular, text, or even image data.
Accenture reports that even brain MRIs will soon be generated synthetically by some organizations, reducing potential security breaches, and enabling more transformative projects given that the data is less restrictive. Generating synthetic data is critical for this use case because the brain structure is unique, and an MRI scan can be used to identify an individual. Therefore, under typical privacy policies, using this identifiable data can be risky and usually would be prohibited or discouraged by organizations. Synthetic data opens the door to opportunities of generating value more easily while mitigating privacy risks.
You will need to strengthen your security measures to demonstrate that the security relative to your material resources, organizations, and techniques is safe in regard to data privacy. A good first step is to document an ISP (information security policy). Then, you might discover irregularities that you will have to manage. Here is a link to some handy templates from SANS.
In conclusion, selecting the right strategy for de-identifying your data is key. Please be careful not to be too restrictive as deleting personal information can restrict the value you can derive from analytics and AI applications. Here is a useful resource from EDUCAUSE to guide you through this exercise.
3. Explicability is becoming a must when building any AI system.
Not only will individuals have the right to understand the reasons behind predictions, but it is also a helpful tool to validate the quality of your AI system.
Are the requirements for explicability restraining organizations from using more sophisticated AI and machine learning algorithms?
No. In fact, over the past decade, the academic community has collaborated to create tools and techniques that generate explanations for potentially very complex algorithms. Nowadays, the challenge comes not from the explainability itself but from explaining the reasons behind the prediction in simple terms. Good User Experience will be required to make the explanations meaningful.
4. Ethical issues and negative bias risk management are other issues that organizations must tackle with C-27.
More concretely, organizations will have to take a risk management approach, which consists of listing potential risks, estimating likelihoods and impacts, and then establishing mitigation plans. This is a simple yet efficient mechanism to manage most risks in an AI project.
To get you started, some actors in the industry have created very useful resources that allow you to complete a self-assessment. Here are 2 useful resources to identify and address ethical and negative bias risks:
- Here is an excellent resource that lists and describes the most relevant risks for an AI system. This work objects to contribute hereto by identifying relevant sources of risk for AI systems. For this purpose, the differences between AI systems, especially those based on modern machine learning methods, and classical software were analyzed, and the current research fields of trustworthy AI were evaluated.
A taxonomy could then be created that provides an overview of various AI-specific sources of risk. These new sources of risk should be considered in the overall risk assessment of a system based on AI technologies, examined for their criticality, and managed accordingly at an early stage to prevent a later system failure. - OBVIA has partnered with Forum IA Québec to create an excellent reflexivity grid on the ethical issues of artificial intelligence systems (this tool is only available in French for the moment). Presented in the form of a questionnaire with open-ended answers, this grid was designed to help team members who design, implement, and manage AI Systems consider the ethical issues arising from the development and use of these new technologies.
This grid is part of a participatory research and aims to develop useful ethical tools for practitioners. It is intended to be constantly evolving in light of the needs and experiences of the actors likely to use it.
I think that self-assessment tools like this one is the way to go as it ensures a certain rigor in the assessment while making the process less painful for end users.
C-27 will come with an extensive and strict set of requirements
In conclusion, C-27 will come with an extensive and strict set of requirements. Although it is for the greater good, organizations will need to put strong effort into their preparations. There are smart ways to be compliant while not jeopardizing your innovation process; purging all your data or not do AI or analytics applications is not a valid option. The silver lining in this situation is that the solutions to comply to C-27 are opportunities to generate additional value.
By controlling your data collection and management process, you will gain maturity, and this should positively impact data collection and quality.
By using de-identification techniques, anonymization techniques only when it is necessary, and by generating synthetic data, you will significantly reduce security risks while pursuing AI applications that seemed too risky before. This will help change management. Synthetic data can also be used to produce larger datasets, which will help build performant AI applications.
By investing in explicability for your AI applications, you will not only comply with C-27 but will also significantly reduce validation and change management efforts as end users and stakeholders can be re-assured when explanations line up with their reality.
Finally, by evaluating and acting upon ethical and negative bias risks, you ensure that your organization does not discriminate against consumers or employees, which can be catastrophic from a legal, reputational, and societal standpoint.
C-27 is good for the population and will help organizations make better use of their data.
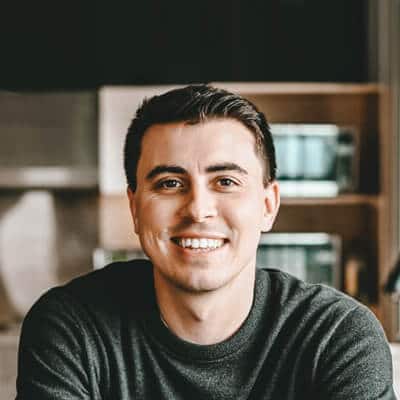
Olivier is co-founder and VP of decision science at Moov AI. He is the editor of the international ISO standard that defines the quality of artificial intelligence systems, where he leads a team of 50 AI professionals from around the world. His cutting-edge AI and machine learning knowledge have led him to implement a data culture in various industries.