Machine learning to facilitate social distancing and minimize the spread of COVID-19 in the Montreal métro.
The Société de transport de Montréal (STM) provides 1.3 million trips a day thanks to a 71-km metro network spread over four lines and composed of 68 stations, combined with 225 bus lines.
It employs more than 10,000 people, making it the 15th largest company in Quebec. The STM provides more than 80% of public transit trips in the Montréal area and more than 70% of all trips made in Québec.
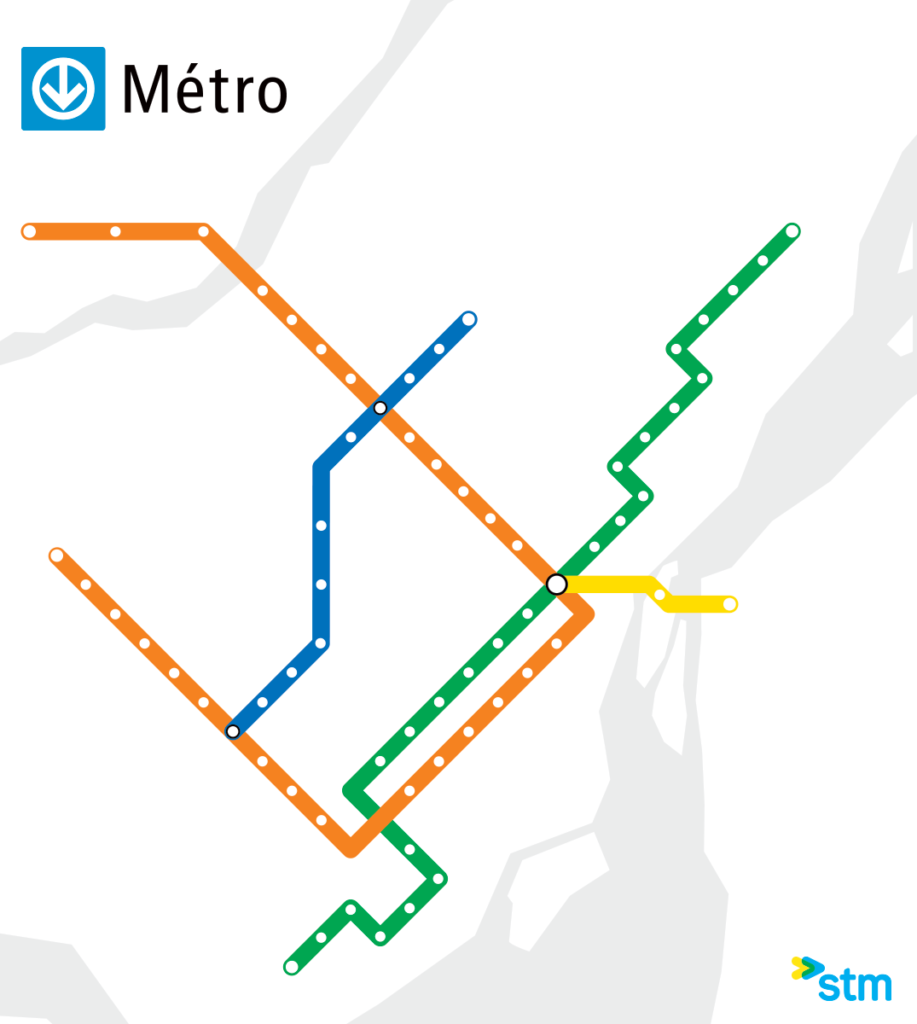
As the city’s backbone, the métro is a prevalent means of transportation and is prized by Montrealers… then came the COVID-19 pandemic in March 2020.
The general confinement decreed by the Government of Quebec, combined with the generalized teleworking that followed for many Montrealers, meant that the métro emptied itself of its passengers.
Like many companies, the STM suddenly lost most of its clientele overnight in its bus and métro network. STM had to react quickly to this new reality and deal with new and immensely complex challenges.
It must now ensure compliance with health rules, such as wearing masks and maintaining a two-meter distance between customers in the métro and bus network.
This social distancing is easier to respect when the métro is empty, but how do you deal with these rules when people return to work during a pandemic? How can we gain users’ confidence while providing tools to ensure their safe return in the métro?
Why not predict traffic in train cars using machine learning to allow customers to move to less busy ones?
In this article
Supporting the technical team in the development of an artificial intelligence model
The STM called upon Moov AI to assist its team in realizing a machine learning project that would predict traffic in train cars to respect social distancing better and thus minimize the spread of the virus in the metro network.
This project aims to inform customers about the number of passengers on the metro’s orange line train cars. This data will be displayed on the website and the Métrovision screens that display information in the metro stations.
The predicted traffic in each train car is displayed, and passengers can position themselves in front of the right doors and choose the less crowded train cars.
We assisted the STM team in their first AI project by helping them overcome the hurdles to deploy a machine learning solution in a cloud service.
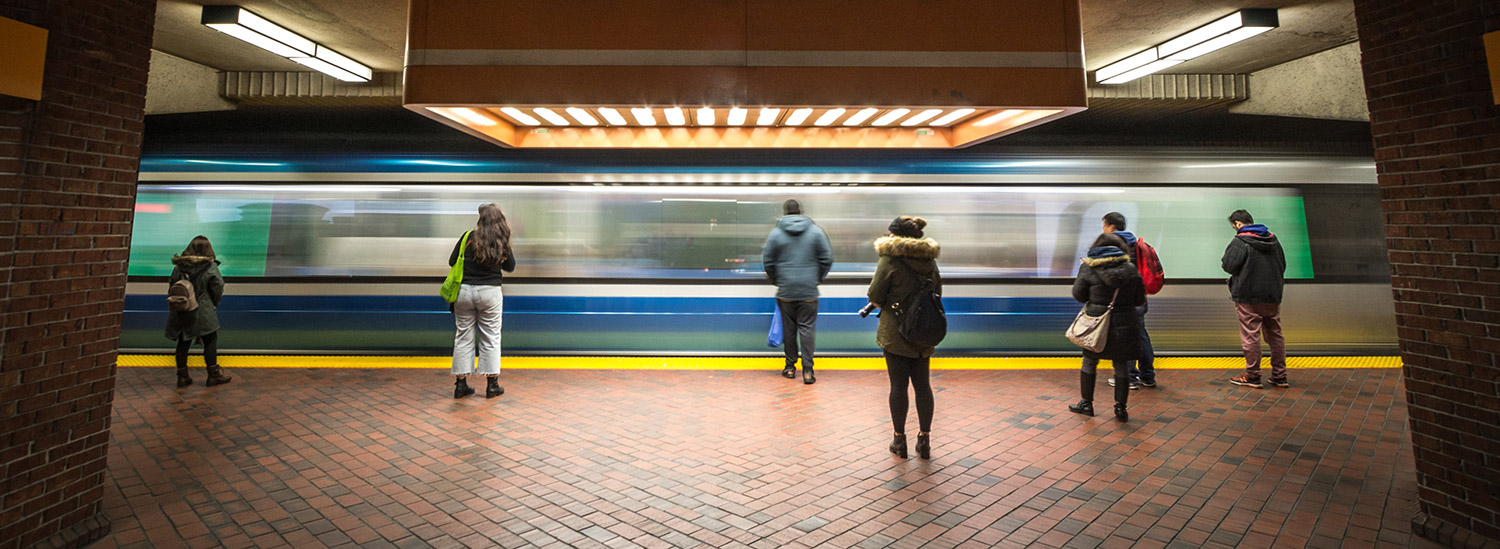
How to calculate traffic
In the solution, the STM used machine learning to predict the number of passengers for each station on the Orange Line, every minute, within a 15-minute horizon.
To build the dataset we needed to make the predictions, the teams used data sources provided by train car telemetry. Azur train cars are equipped with a phenomenal amount of sensors of all kinds that provide data every minute.
The solution will use load sensors that calculate the cars’ weight to evaluate the number of passengers. Logically, a heavier car will have more passengers. This data is very accurate and allowed to reach an adequate margin of error for the first project.
Overcoming the obstacles to putting a first AI project into production
We guided STM’s data scientists and data developers get over the hurdles of deploying the first version of their solution in the cloud. The project was a success, and then some…
Using these learnings, their team deployed a new version of the AI model based on real-time data from the Orange Line cars even better to predict traffic on the metro.
This new AI system is displayed on the screens of your favorite stations on the orange line.
We are proud to have accompanied the STM teams who have restored the confidence of Montrealers using the metro to return to the underground facilities in complete safety.
Democratizing AI in organizations requires, among other things, the empowerment of our clients’ teams.
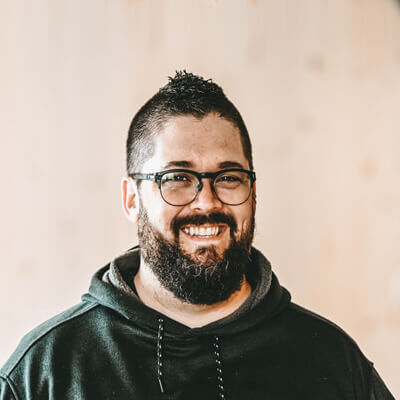
Guillaume is co-founder and VP Marketing of Moov AI. A natural communicator, he is dedicated to promoting the brand and growing the company. He prides himself on forging solid partnerships and developing strategies to ensure optimal strategic positioning for Moov AI. As a speaker, Guillaume enjoys awakening audiences to the transformative influence of AI in business ecosystems. He has extensive marketing expertise in different industries, having held the position of Marketing Director at GSoft (Workleap) and Pyxis Technologies.