Generative artificial intelligence: why is everyone talking about it?
With the growing popularity of generative artificial intelligence technologies, such as PaLM 2 and ChatGPT, more and more companies are seeking ways to integrate AI into their daily operations. According to a McKinsey report, generative AI will have a significant impact on the economy by increasing the economic value of AI by 15 to 40%, representing an estimated annual value of $2.6 to $4.4 trillion. That’s huge!
Generative AI has the potential to revolutionize several sectors, and currently, generative AI solutions are already being deployed to simplify tasks and optimize processes. Google and Microsoft now offer tools specifically designed to facilitate the integration of this technology in enterprises. By the way, if you haven’t already read it, we recommend using generative AI tools in workplaces by opting for a solution tailored for businesses.
In short, generative AI offers the potential to automate, improve, and accelerate various tasks. In this article, our goal is to explore how this technology can enhance work and demonstrate how companies can benefit from it.
In all the examples below, we advise, as with all AI systems we develop, involving humans in the process. For us, generative AI improves the efficiency of your employees, but it is essential to keep humans in the loop.
Without further ado, here are a few examples.
Use of Generative AI
1. Content Generation
Generative AI opens up impressive new perspectives in the realm of dynamic content creation. A well-known use case, generative AI can be employed to automatically generate text. This technology can be applied in various contexts, here are the most relevant ones:
- Generating Metadata for Products.
Generative AI is revolutionizing how businesses manage product metadata in their inventory by automating tagging, description creation, and categorization. Through advanced natural language processing and image analysis, generative AI extracts essential attributes of products, generating accurate and relevant metadata. These operations optimize stock update processes and also enhance search functionality and user experience on e-commerce platforms. The scalability and efficiency of generative AI make it an invaluable tool for companies seeking to optimize their product information management. - External Conversational Agent.
Conversational agents or chatbots powered by generative AI can interact with users in a natural and seamless manner while adhering to internal governance policies and your brand image. They can generate relevant and coherent responses based on posed questions, thereby improving user experience and customer service efficiency. - Document Generation.
Generative AI can produce comprehensive documents such as reports, blog articles, summaries, etc., based on provided input information. This can be particularly useful for generating extensive content, for example, legal reports and case analyses for lawyers.
2. Text Summarization
Generative AI’s ability to distill the essence of a text and summarize it concisely finds diverse applications:
- Customer Flow Analysis.
By analyzing customer comments, reviews, and reactions, generative AI can generate summaries that provide valuable insights into customer trends and preferences, thus helping businesses make informed decisions. - Research Assistance for Experts.
In technical or specialized fields, generative AI can assist experts by generating summaries of complex research or condensing technical documents into understandable key points. For instance, in the banking sector, generative AI can play a crucial role in supporting experts in understanding and interpreting complex research related to finance, economics, and markets. A concrete example would be analyzing and synthesizing detailed financial reports and academic research papers. - Item Segmentation into Categories.
Generative AI can aid in segmenting large amounts of text into relevant categories, which is useful for organization and subsequent data analysis. In marketing, companies often collect vast amounts of data from various sources, including social media, surveys, and market analyses. Generative AI can be used to segment this data into relevant categories. For instance, a fashion company can use AI to classify customer comments based on style trends, color preferences, or reactions to different collections. To facilitate inventory management, businesses can segment items, stores, or customers using structured data. Generative AI rapidly identifies dominant customer opinions and behaviors, enabling better decision-making while maintaining effective stock management.
Series on generative artificial intelligence
This article is part of a series we have produced to help businesses better understand generative AI and its possibilities.
- Solutions for SAP, Oracle, and Microsoft Dynamics Inventories
- Why you should use ChatGPT in a business context
- How to leverage generative artificial intelligence solutions in business without drifting [Conference on demand]
3. Generating Multiple Content Types
Generative AI is transforming the creation of computer code and software solutions:
- Code Generation (Text-to-Code Conversion).
By understanding natural language instructions, generative AI can convert functional specifications into source code, thereby accelerating the development process. - Image Personalization.
Generative AI can create customized images based on textual descriptions, offering new possibilities for visual customization. In product design, design teams can quickly and automatically explore different visual variations of a product based on textual specifications. This can expedite the prototyping process and allow for the exploration of visual concepts before materializing them. - Recommendation Engine.
Generative AI excels in creating tailor-made code recommendations and software architectures. This results in enhanced development team efficiency, enabling rapid detection of code anomalies and instant receipt of improvement suggestions.
4. Semantic Research
Generative AI is an asset in complex data research and analysis:
- Internal Conversational Agent.
Organizations can benefit from internal conversational agents that assist employees in quickly searching for and retrieving information from vast databases, including their internal database. Employees can interact with the agent naturally to ask complex questions and receive relevant answers, facilitating decision-making and access to internal knowledge. - Insight Generation.
Generative AI can help identify trends and hidden insights in large and diverse datasets, offering a fresh perspective on research. This can be useful for analyzing unstructured data, identifying trends, creating customer segments, or predicting future trends. This capability allows businesses to rapidly extract impactful information from documents and transform them into actionable knowledge. - Customer 360° View.
Generative AI can be used to aggregate and unify heterogeneous data into a comprehensive view of each customer. Using advanced machine learning and natural language processing techniques, AI can identify relationships between different data points and create enriched customer profiles. This enables sales, marketing, and customer service teams to have a deep understanding of each customer’s preferences, behaviors, and needs.
Essential Use Cases of Generative Artificial Intelligence
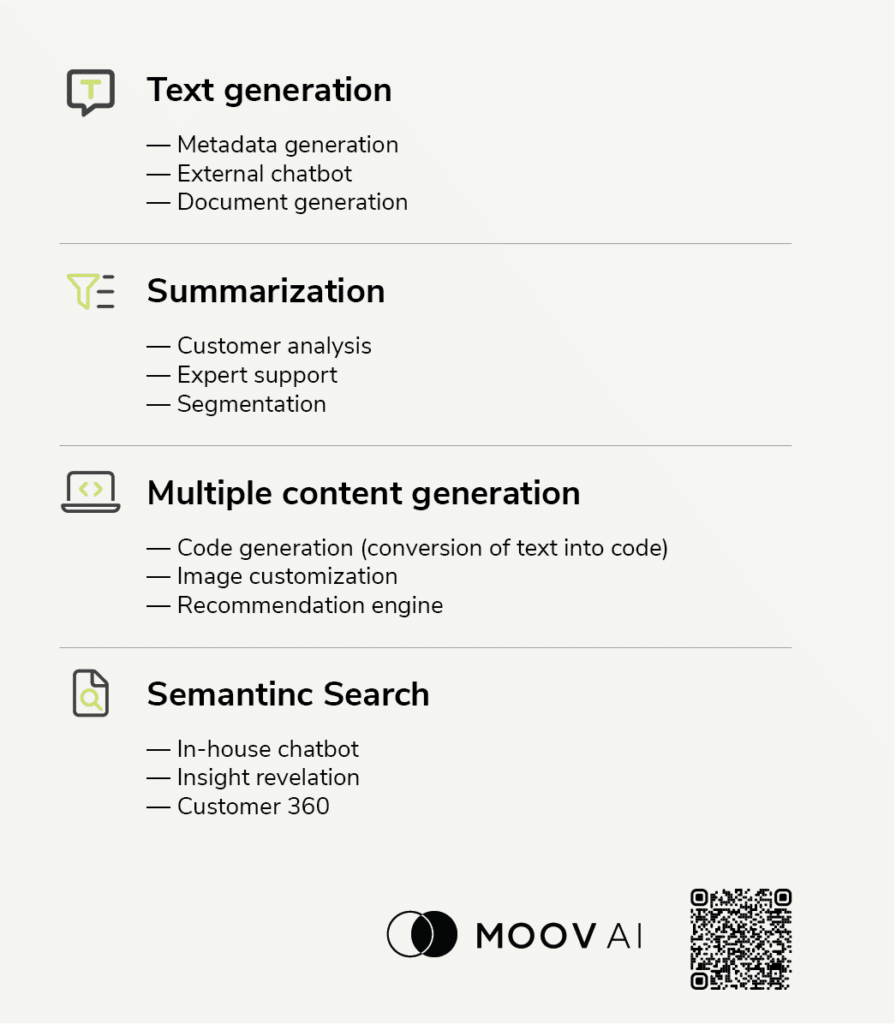
[Cheat sheet]
Essential use cases for generative artificial intelligence
Download our Generative AI use case checklist. Simply fill in the form and you’ll receive your copy by e-mail.
Initiate by selecting a low-complexity, high-value use case for your organization
When embarking on a generative AI project, it’s often advisable to begin with a Proof of Concept (PoC) that offers low complexity and rapid high value. A PoC is a practical demonstration that validates the technical feasibility and potential value of a solution before committing to a fully integrated system.
Let’s consider the concrete example of a Proof of Concept for a generative AI-powered virtual assistant. Such a system enables customer support agents to easily access internal knowledge sources, ask questions, and receive relevant real-time answers. Swiftly showcasing the power of such a solution on your data and within your corporate context can not only enhance employee productivity but also generate enthusiasm by highlighting the benefits of generative AI within the organization.
Furthermore, through an internal virtual assistant PoC, a company can test the effectiveness of generative AI before applying it to customer-facing applications. This helps comprehend limitations and necessary improvements while minimizing the risks associated with implementing new technology.
“With great power comes great responsibility.”
-Uncle Ben
At Moov AI, we believe in the immense potential of generative artificial intelligence and advocate for a more responsible use of AI through the leadership of Olivier Blais in LIAD, ISO standards on AI, and with the Quebec Innovation Council. Just like with any AI project, we aim to reduce risk levels. You can watch Oliver’s conference on generative AI, where the risks associated with this type of project are addressed along with how to mitigate them. It’s crucial to maintain cautious optimism. While the technology is impressive, it needs to be explored with security at the forefront.
In conclusion
In an environment where the possibilities for using generative AI are vast, it’s essential to be discerning when choosing which use cases to explore. Before adopting a generative AI solution, as with all AI projects, it’s vital to think about the business objectives you’ve set yourself. An AI solution must meet your business objectives.
Now that we’ve outlined the various use cases for generative AI, you need to ask yourself what the next steps are. The first thing we’d advise you to do is to consider the questions proposed by McKinsey.
- To what extent can technology help or disrupt our industry and/or our company’s value chain?
- What are our policies and positions? For example, do we wait cautiously to see how the technology develops, invest in pilot projects, or seek to develop a new business? Should the position vary according to areas of the business?
- Given the limitations of the models, what are our criteria for selecting which use cases to target?
- How do we go about creating an effective ecosystem of partners, communities and platforms?
- What legal and community standards must these models comply with so that we can maintain the trust of our stakeholders?
If you want to know more about the best way to start an AI (or generative AI) project, we’ve got a good eBook for you.
Getting started with a proof of concept can be a beneficial approach, offering quick value while enabling your organization to become familiar with generative AI and develop internal traction in the face of innovation. By taking these preliminary steps, you’ll be better prepared to maximize the benefits of this emerging technology, while addressing the specific needs of your business.
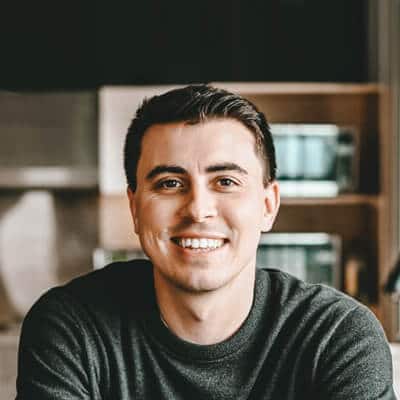
Olivier is co-founder and VP of decision science at Moov AI. He is the editor of the international ISO standard that defines the quality of artificial intelligence systems, where he leads a team of 50 AI professionals from around the world. His cutting-edge AI and machine learning knowledge have led him to implement a data culture in various industries.