Imagine a scenario where the descriptions and categories of your inventory products are generated automatically. This prospect is now within reach thanks to generative artificial intelligence.
Like any innovation project, the aim is to achieve increased productivity, reduced costs, better resource management, all with the ultimate goal of gaining a competitive advantage. And this is precisely what the integration of AI into the ERP enables.
The use case presented in this article, namely metadata generation, is unquestionably an opportunity for businesses in the retail sector that utilize ERPs like SAP, Oracle, and Microsoft Dynamics.
In This Article
Benefits of Integrating Generative AI with SAP / Oracle / Microsoft Dynamics
Improved Search and Recommendations
Thanks to precisely generated metadata, search and recommendation functionalities within the ERP can be greatly enhanced. For instance, AI-driven search could yield more pertinent results for users seeking specific products or components
Data Enrichment
Beyond basic metadata, generative AI can also contribute to enriching product data. For example, it can suggest potentially complementary products or add-ons based on metadata from other similar products.
Scalability
For companies with vast and constantly evolving inventories, manually updating or creating metadata for each product can be a challenging task. Generative AI can be scaled up to handle thousands of products, ensuring consistent metadata generation and updates.
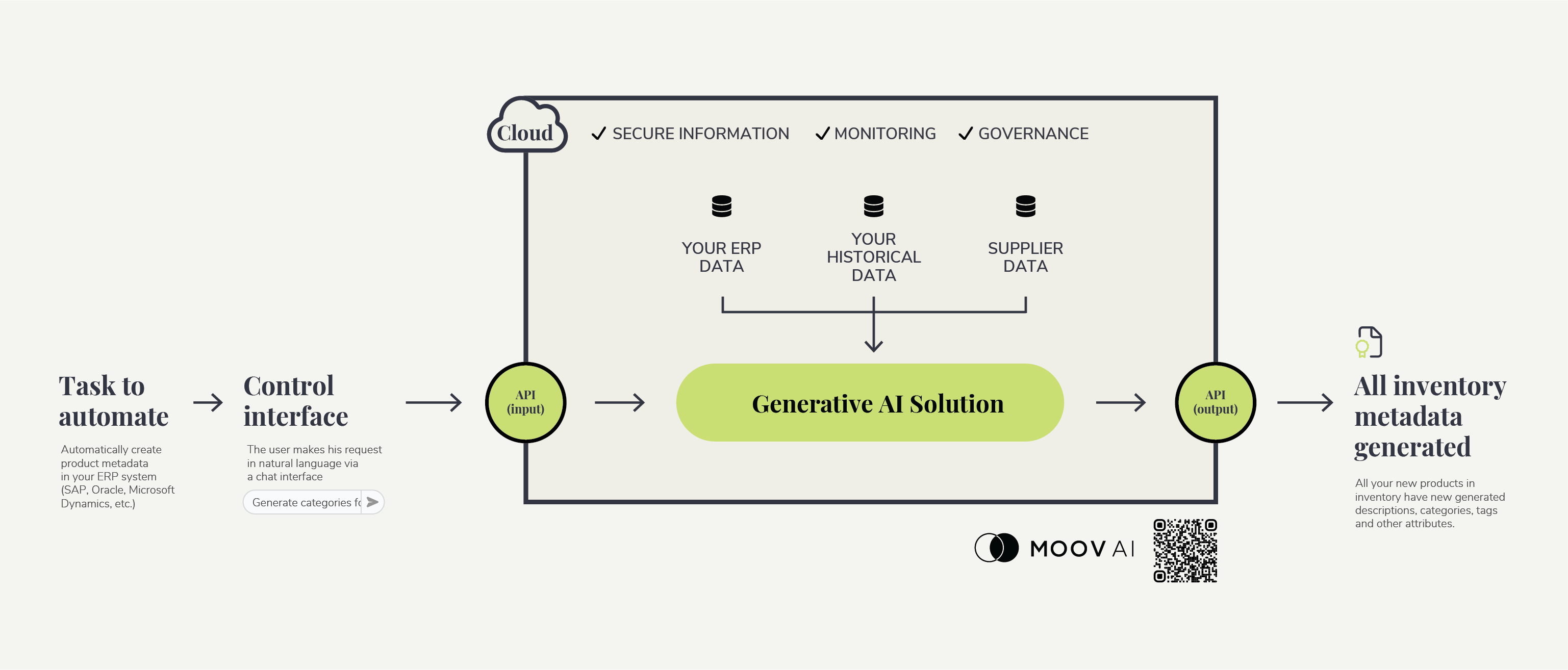
Operation of Generative AI and Integration into SAP / Oracle / Microsoft Dynamics
Metadata generation from text
Metadata generation from an image
1. Metadata Generation
Automated Generation of Descriptions
For new products, AI automatically generates descriptions based on similar products in the inventory or on brief information provided by the user.
Categorization and Labeling
Generative AI can suggest or generate categories or labels for products based on their descriptions, images, or other attributes.
Localization
If you operate in multiple regions, AI can be trained to generate product metadata in several languages, facilitating the localization of inventory items.
2. Quality Control and Refinement
Feedback Loop
To continually enhance accuracy, a feedback mechanism is implemented where incorrect or inadequate metadata generated by AI is corrected by humans. These corrected data points serve as additional training data, refining the AI’s results over time.
Validation Process
Before newly generated metadata is accepted, a validation step is conducted to ensure accuracy and relevance.
3. Integration with the ERP
The generative AI system can be integrated with SAP, Oracle, or Microsoft Dynamics. This can be achieved through API integrations or custom modules, ensuring that the generated metadata seamlessly integrates into the inventory management system.
Series on generative artificial intelligence
This article is part of a series we have produced to help businesses better understand generative AI and its possibilities.
- The Essential Use Cases of Generative Artificial Intelligence
- Why you should use ChatGPT in a business context
- How to leverage generative artificial intelligence solutions in business without drifting [Conference on demand]
Metadata Generation with Artificial Intelligence [Video featuring Olivier Blais] (French)
In this video, I delve into how generative AI provides businesses working with solutions like SAP, Oracle, and Microsoft Dynamics the opportunity to streamline the automatic generation of metadata for their inventory products. Applying this technology to this specific context undoubtedly stands as one of the most promising prospects in the realm of retail. I wish you an enjoyable viewing experience.
[This article is a verbatim translation of the video segment by Olivier Blais, generated by generative artificial intelligence tools and corrected by a human.]
Introduction to Generative AI for Metadata Generation
Hello everyone. This week, we’re going to discuss a use case of generative artificial intelligence that particularly interests me. Why? Because it’s a case that will truly save time and help us address a rather tedious issue, which is creating metadata.
I’m not sure if you’re aware, but for a store to display thousands of products on a website, for example, or to ensure that all products, all items are properly cataloged, it takes a lot of manual effort, a lot of time, and requires large teams to enter information into systems. Often, this information is duplicated, and sometimes it involves taking information from one system and converting it to be used in another system. Sometimes, it’s about analyzing a product description to be able to categorize it. And this is a requirement, something we always need, whether it’s in ERP-style systems or all sorts of other systems, and it’s a lot of work that’s needed.
Here, from the beginning, for years, we’ve been saying that we’ll try to do things as best as we can. So, we end up creating processes that are a little more efficient. We save minutes here and there by simplifying the metadata generation process.
Opportunity in Retail
However, with generative AI, we bring solutions that will change how we generate our information. Here, all we need is to have a product description, for example, and to know what the different fields are, what the requirements are, a few constraints to be able to generate metadata very precisely.
We’ll not only be able to save time, but also increase speed. We might see a reduction of fifty to seventy-five percent in the time it takes to generate metadata, but we’ll also gain in accuracy. We’ll be able to generate information of much higher quality.
How We Generate Metadata
How does this work? It’s based on the metadata we already have in our systems. So here, we don’t even need to leave our systems. We take information we already have and draw inspiration from it. We take these examples and provide them to the generative AI.
So, we take a product description, we take examples of metadata, and essentially say to replicate this structure. And there you have it.
Practical Application of AI for Metadata Generation
In fact, here’s an example. Let’s take a hardware store or a grocery store, for instance. Consider a grocery store; it has a lot of inventory items.
You go to the grocery store, there are tens of thousands of items. And every month, every week, there are even new items. So, what that means is that every week, you potentially have a team of a hundred people whose task is to enter information about a new type of tomato or new cans of soup in order to properly catalog and sell them. This is tedious, time-consuming, and doesn’t add much value.
What we’re talking about here is essentially taking information provided by suppliers, entering it into a generative AI solution, and getting back the necessary metadata to enter into an ERP solution like SAP. Once the metadata is in the SAP solution, the job is done, and you can start selling the item and put it on the shelves.
Feel free to share if you have other ideas for use cases; I’d be happy to discuss them.
Conclusion and Perspectives
In conclusion, the integration of generative artificial intelligence into business practices paves the way for significant and transformative advancements.
The approach to metadata generation relies on information already present in the company’s systems, thus avoiding unnecessary efforts and redundancies. By simply providing a product description and examples of metadata, companies can swiftly and accurately obtain the data needed to fuel their ERP systems. This innovation simplifies and streamlines inventory management, allowing teams to focus on higher-value tasks and accelerating the introduction of new products to the market.
The use case in the retail sector is just the beginning of a broader exploration of the possibilities offered by generative artificial intelligence.
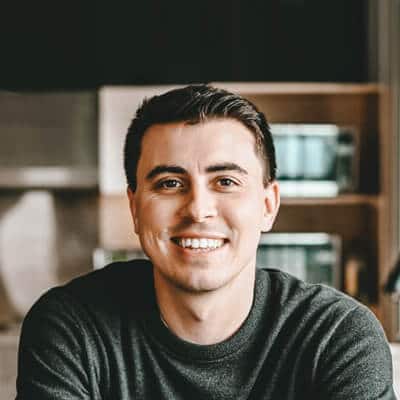
Olivier is co-founder and VP of decision science at Moov AI. He is the editor of the international ISO standard that defines the quality of artificial intelligence systems, where he leads a team of 50 AI professionals from around the world. His cutting-edge AI and machine learning knowledge have led him to implement a data culture in various industries.