Anticipating future trends is the key to success for retail, food, and consumer goods (CPG) companies. And when it comes to prediction, artificial intelligence (AI) is emerging as the super fuel for this forecasting machine.
Nothing is forever except change.
Bouddha
Managers dealing with seasonality, sudden changes in demand levels, events, supplier price fluctuations, strikes, and economic upheavals don’t view forecasting tools as a luxury but as a necessity for making informed decisions.
And it’s understandable. The impact of poor decisions made “blindly” or with inaccurate predictions can far outweigh the cost of integrating AI to obtain precise forecasts.
This article explores how retail, consumer goods, and food companies can enhance their decision-making through artificial intelligence. We will highlight the potential benefits through concrete examples of usage.
From Data to Benefits
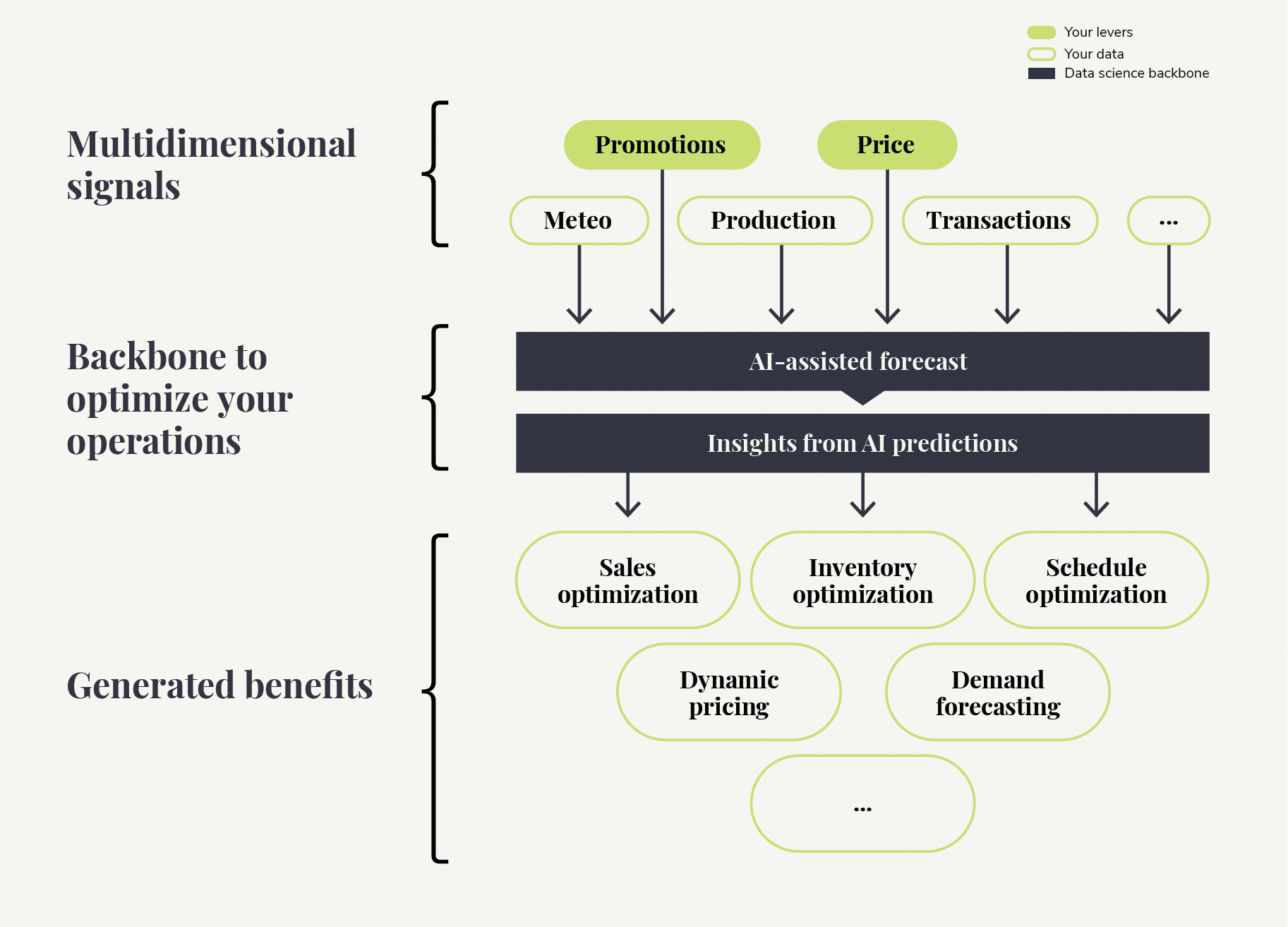
The artificial intelligence solution serves as the engine of the model, enabling the extraction of insights and, ultimately, facilitating better decision-making based on these insights. Data is the fuel, and AI is built on observed data, making predictions extremely reliable and highly accurate.
Multidimensional Signals
Simultaneously evaluating the impact of multiple variables is an extremely complex, if not impossible, task for humans. AI is capable of detecting variables that have a real impact on predictions and quantifying their influence on your business. Among these variables, referred to as “multidimensional signals,” are meteorological data, production data, transaction data (POS), current and upcoming promotions, sales prices, essentially all data that can impact demand.
Some of these variables are designated as “levers,” such as price and promotions. These are variables over which the company has complete control (unlike the weather, which would be convenient, though) and with which it is possible to conduct simulations to determine optimal pricing or even implement dynamic pricing.
AI-Powered Forecasting: The Backbone of the Solution
AI-driven forecasting supports all the previously mentioned data and automatically integrates it into the AI model in an automated process. This AI model can identify complex patterns within the data, thereby discerning true trends and generating crucial insights for decision-making.
In the retail, food, and consumer goods sectors, forecasting goes far beyond simple predictions. It represents an essential tool for anticipating future trends, optimizing operations, and maintaining competitiveness.
Here is an example of the precision achievable in your forecasts through AI
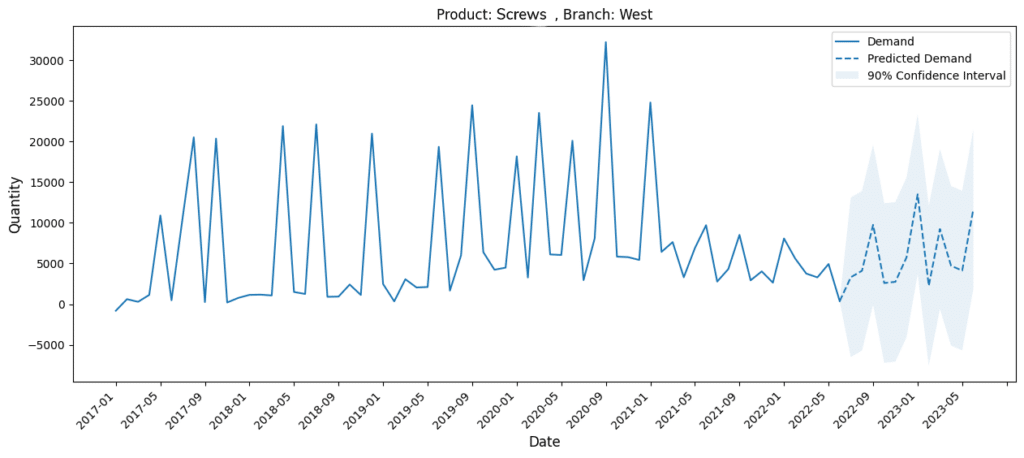
In this example, the precision of AI allows us to forecast future demand for screws at a retailer, within a 90% confidence interval.
The Benefits
By deriving insights, we gain a better understanding of the situation, enabling us to make more informed decisions and optimize our operations. For a simple example, with information like the correlation between a sunny day’s weather, the ongoing promotion on hot dog buns, and historical sales data, we can accurately predict the right quantity of hot dogs. In this simple use case, we achieve:
- Optimizing inventory management
- Optimizing schedules by knowing the quantity of items expected to be sold
This, in turn, allows us to meet your assumptions, maximize operational efficiency, profitability, all while reducing your team’s workload.
The Complete List of AI Use Cases in Retail
In this section, we will explore various concrete use cases of forecasting with artificial intelligence in detail, demonstrating how this innovative technology can fundamentally transform how companies approach demand, production, inventory management, and more.
Using real-world examples and inspiring case studies, we will discover how AI is redefining the standards for strategic and operational planning.
Without further ado, here is the list of all use cases. Use this article as a reference, using the links below, which will take you to a description of each use case.
List of Use Cases for Artificial Intelligence in Retail, Consumer Goods, and Food Industry.
- Metadata Generation for Inventory Products
- Demand Forecasting
- Stock Optimization
- Production Planning Based on Demand
- Price Optimization
- Dynamic Pricing
- Price Elasticity Analysis
- Personalized Pricing
- Promotional Pricing Optimization
- Competitive Pricing Analysis
- Supply Chain Management
- Transport Demand Forecast
- Production Capacity Planning
- Lead Time Forecasting
- Shipment Delay Forecast
- Demand Variability Analysis
- Stock Optimization
- Supplier Performance Forecast
- Promotion Optimization
- Demand Shaping
- Channel-Specific Promotions
- Competitive Analysis
- Seasonal and Event-Based Promotions
- Demand Variability Analysis
- Assortment Planning / Market Basket Analysis
- Sales Forecast
- Workforce Management
1. Automatic Metadata Generation with AI

To begin, this is a use case of generative artificial intelligence that revolutionizes metadata management by automating the creation of product labels and descriptions. Integrated into existing systems, it ensures consistency in line with the company’s standards. Leveraging advanced natural language processing and image analysis, it generates precise and relevant metadata.
In practice, the current metadata structure of the company is used as an example to provide to the generative AI model. With descriptions of future products, the model generates new metadata following the same logic.
This simplifies inventory management and enhances the user experience on e-commerce platforms. I delve into this use case in-depth in a video segment within this article. In my opinion, it brings significant value through cost savings while improving task efficiency.
2. Demand Forecasting

Un système d’IA bien conçu pour aider les gérants en magasin à commander la bonne quantité de marchandises qui seront vendues dans les prochains jours ou semaines. C’est ce que nous avons fait pour les épiceries Métro et leur demande en produits périssables.
A well-designed AI system to assist store managers in ordering the right quantity of goods that will be sold in the upcoming days or weeks. This is precisely what we’ve accomplished for Metro grocery stores and their demand for perishable products.
3. Stock Optimization

By accurately forecasting demand, companies can avoid stockouts (and reduce excess inventory). This helps improve customer satisfaction, minimize holding costs, and effectively manage the supply chain.
- Demand-Driven Stock Planning: Accurately predict future demand to optimize stock levels by stocking the right quantity of products at the right time, thus reducing the risk of stockouts or overstock.
- Dynamic Replenishment: Adjust stock levels dynamically to minimize stockouts and avoid overstock.
- Seasonal Demand Planning: Adapt supply, production, and distribution plans to ensure adequate stock availability without excessive holding costs.
- Just-in-Time Inventory Based on Demand Forecasting.
4. Demand-Driven Production Planning

Manufacturers and suppliers rely on demand forecasts to plan their production schedules and allocate resources efficiently.
By understanding future demand trends, they can adjust production capacity, optimize raw material procurement, and streamline manufacturing processes to meet the anticipated needs of customers.
- Demand-Centric Production: Adjust production capacity and align manufacturing processes to meet anticipated customer demand.
- Raw Material Procurement: Forecast demand to adjust procurement schedules, ensure timely availability of materials, and avoid stockouts or excess inventory.
- Production Planning for Seasonal Variations: Provide insights into expected demand during specific seasons to adjust production schedules, allocate resources appropriately, and optimize stock levels to meet seasonal demand fluctuations.
5. Price Optimization

The fifth application stands as a key element for maximizing revenue while ensuring customer satisfaction and competitiveness in the market. The use of AI in forecasting allows for precise price optimization.
- Dynamic Pricing: Dynamically adjust prices based on demand fluctuations, competitor behavior, or other market conditions to optimize real-time pricing, maximize revenue, and respond more effectively to market dynamics.
- Price Elasticity Analysis: Understand price elasticity, which measures the sensitivity of customer demand to price changes, to determine optimal price points that balance revenue maximization and maintaining customer demand. This enables setting prices that achieve the highest possible value without sacrificing sales volume.
- Personalized Pricing: Segment customers based on their preferences, purchasing behavior, or other relevant factors to generate tailored pricing recommendations for each customer segment. Companies can offer personalized prices or discounts that match individual customer needs and enhance customer loyalty.
- Promotional Pricing Optimization: Generate insights into the most effective promotional pricing strategies to determine the right discount levels, duration, and timing of promotions to maximize sales and profitability.
- Competitive Pricing Analysis: Understand competitors’ pricing strategies to adjust your own pricing strategies to remain competitive while preserving profitability.
6. Supply Chain Management

AI-powered forecasting enables accurate demand forecasting, efficient inventory management, streamlined production, and effective logistical planning, leading to a more agile and resilient supply chain.
Improving supply chain planning reduces stockouts and overstocks and enhances overall operational efficiency.
- Transport Demand Forecasting: To optimize logistical planning, allocate the appropriate transport resources, and enhance delivery efficiency. Accurate forecasts enable better coordination with carriers and reduce transportation costs.
- Production Capacity Planning: To optimize production planning, ensure optimal resource utilization, and avoid capacity constraints. Precise capacity forecasting improves production scheduling and minimizes disruptions.
- Lead Time Forecasting: To forecast lead times for raw materials or finished products, optimizing inventory levels, production scheduling, and managing customer expectations. Accurate lead time forecasting enables better inventory replenishment and reduces stockouts or excess inventory.
- Shipment Delay Forecasting: To predict potential shipment delays proactively manage potential disruptions, adjust production or inventory plans, and communicate timely updates to customers. Accurate shipment delay forecasting improves customer satisfaction and supply chain reliability.
- Demand Variability Analysis: To understand the extent of demand fluctuations, plan safety stock levels, and adjust inventory strategies to mitigate the impact of demand uncertainties. Precise demand variability analysis minimizes storage costs and stockouts.
- Inventory Optimization: To optimize stock levels in storage facilities based on product demand and inventory turnover. Accurate storage level forecasting allows for efficient inventory management, reduces inventory costs, and minimizes financial commitments related to stock.
- Supplier Performance Forecasting: To evaluate supplier reliability, identify bottlenecks or risks, and optimize supplier selection for improved supply chain efficiency.
7. Promotion Optimization

AI models can evaluate the effectiveness of different types of promotions, schedules, and discount levels. By understanding the impact of promotions on demand, businesses can optimize their promotional strategies, allocate resources efficiently, and ensure sufficient stock levels to meet increased demand during promotional periods.
- Demand Shaping: Predict the potential impact of different promotional scenarios on customer demand. By simulating various promotional strategies, price discounts, or product bundling options, AI models can help retailers and consumer goods companies shape customer demand and optimize promotional plans. This improves stock planning, production scheduling, and resource allocation.
- Channel-Specific Promotions: AI forecasts can analyze customer preferences, buying behavior, and channel-specific data to optimize promotional strategies for different sales channels. Tailored promotions suited to the unique characteristics of each channel can be recommended. This helps retailers and consumer goods companies offer personalized experiences and boost sales through the most effective channels.
- Competitive Analysis: AI forecasts can analyze competitor promotional data, market trends, and pricing information to provide insights into competitive promotional strategies. By monitoring and evaluating competitor promotional activities, AI models can help retailers and consumer goods companies stay competitive, adjust prices and promotional tactics, and effectively gain market share.
- Seasonal and Event Promotions: AI forecasts can analyze historical sales patterns, customer behavior, and external events to optimize seasonal or event-based promotions. By understanding the impact of specific seasons, holidays, or cultural events on customer demand, AI models can recommend optimal promotional strategies and schedules. This allows retailers and consumer goods companies to maximize sales opportunities during peak periods.
- Assortment Planning/Market Basket Analysis: This is a strategic process in the retail and consumer goods sectors that involves determining the optimal range and mix of products to offer to customers. The goal is to create a well-designed product assortment that matches customer preferences, maximizes sales potential, and enhances overall customer satisfaction. Discovering relationships between products frequently purchased together allows for cross-selling and upselling opportunities. This optimizes product placement, assortment planning, and targeted marketing campaigns to maximize revenue.
8. Sales Forecasting

Businesses can make informed decisions about sales targets, resource allocation, and marketing strategies based on accurate forecasts powered by artificial intelligence.
Ultimately, this results in precise demand forecasting, better sales planning, improved inventory management, and more. This, in turn, provides a competitive advantage.
- Customer Segmentation: Segmentation of customers to forecast sales and inventory, create marketing campaigns, and optimize operations based on the most performing segments.
- Category-Specific Demand Forecasting: Provides insights into demand patterns and trends for different product categories to generate accurate forecasts for specific product categories. This enables retailers and consumer goods companies to optimize stock levels, adjust production plans, and plan marketing strategies based on anticipated demand for each category.
9. Workforce Management

AI enables the optimization of workforce planning, efficient scheduling, and skills-based allocation, ultimately leading to improved productivity, reduced labor costs, and an enhanced overall experience for employees and customers.
- Demand-Based Scheduling: Aligning staffing levels with anticipated demand to ensure adequate coverage during peak hours and minimize labor costs during slower periods. This maximizes productivity and reduces labor inefficiency. It involves matching staff skills and expertise to specific tasks or professional requirements.
- Staff Allocation: Understanding demand fluctuations to efficiently allocate the workforce to areas requiring more support, optimizing labor resources and improving operational efficiency.
- Seasonal Workforce Planning: Forecasting future workforce needs. This allows for proactive hiring, training, and planning for temporary or seasonal workers, ensuring adequate staffing levels during peak periods.
- Training and Skill Development: By providing predictive tools that aid decision-making, Metro has been able to train new employees more quickly to fulfill orders.
Where to Begin?
In an environment with vast possibilities for utilizing artificial intelligence, it’s essential to exercise discretion when choosing use cases to explore.
Before adopting an AI solution, it’s crucial to reflect on the business objectives you’ve set. An AI solution should align with your business goals.
Next, I prioritize Proof of Concept (PoC) to reduce complexity and quickly obtain high value. A PoC is a practical demonstration that validates the technical feasibility and potential value of a solution before committing to a fully integrated solution in your systems. If you want to learn more about the best method for starting an AI project, we have a helpful eBook for you.
In Conclusion
Store managers must juggle a variety of dimensions, products, and data sources. Predicting the demand for each item and converting it into the quantity to order to achieve an optimal balance between increased sales and reduced losses is an extremely challenging task.
Handling this considerable amount of data surpasses the capabilities of the human brain. This project faithfully embodies our vision of “HumanAI”: a tool powered by AI that enhances individuals’ capacity to make better decisions.
Artificial intelligence is revolutionizing how businesses in the retail, food, and consumer goods sectors approach forecasting. With its advanced data processing and machine learning capabilities, AI offers the ability to predict accurately, plan efficiently, and proactively innovate.
It’s no longer merely an option but a necessity for businesses aspiring to thrive in a competitive and ever-evolving commercial environment.
Q&A Section:
How does AI differ from traditional forecasting methods?
AI differs from traditional forecasting methods by leveraging machine learning and analyzing extensive datasets to identify patterns and make precise predictions. This eliminates human biases and reduces errors commonly associated with manual analysis.
What are the advantages of AI forecasting in the retail industry?
Some advantages of AI forecasting in the retail industry include increased accuracy, improved efficiency, real-time insights, and demand optimization. These benefits help retailers optimize their operations, reduce costs, and deliver superior customer experiences.
What challenges are businesses likely to face when implementing AI forecasting?
Businesses may encounter challenges related to data quality and accessibility, model interpretability, and ongoing monitoring and adaptation. To overcome these challenges, having adequate infrastructure, interpretability techniques, and regular updates is necessary to ensure the accuracy and effectiveness of AI forecasting models.
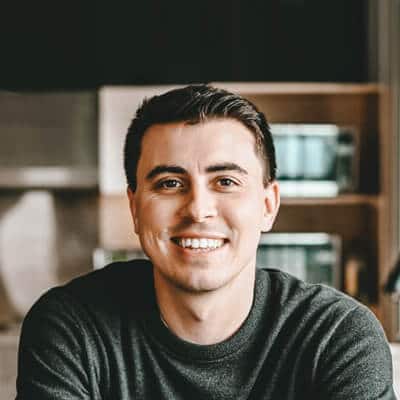
Olivier is co-founder and VP of decision science at Moov AI. He is the editor of the international ISO standard that defines the quality of artificial intelligence systems, where he leads a team of 50 AI professionals from around the world. His cutting-edge AI and machine learning knowledge have led him to implement a data culture in various industries.