AI system quality at Moov AI, a quick guide
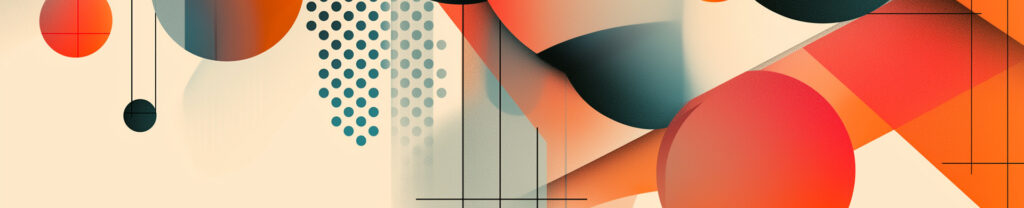
Let’s get straight to the point: evaluating your AI systems is a necessity. It’s as simple as that. We’ve chosen to implement a framework for assessing the quality of AI systems in our projects, based on my work over the last few years with ISO standards. But don’t worry, it’s not black magic, nor is […]
Using artificial intelligence as a health and safety analysis tool in your industry
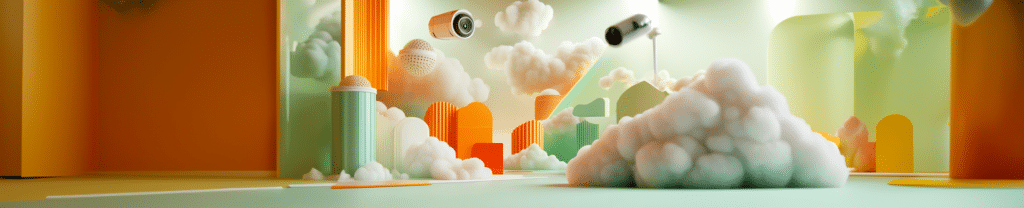
Warehouses are areas where health and safety risks can be high due to the presence of heavy machinery, chemicals, and material handling activities. To improve worker safety and ensure regulatory compliance, using artificial intelligence (AI) to monitor and detect health and safety issues from surveillance footage becomes a solution. These systems have the ability to […]
Optimize operations: generate the right task list for your employees from photos

With labor shortages on the rise and fewer and fewer hours available to get the job done, you need to make sure your employees are efficient and productive… and working on the right things in the right order. Efficient task management is essential, and generative artificial intelligence (AI) is one solution for optimizing your day-to-day […]
Use cases for generative AI that will be really useful in 2025
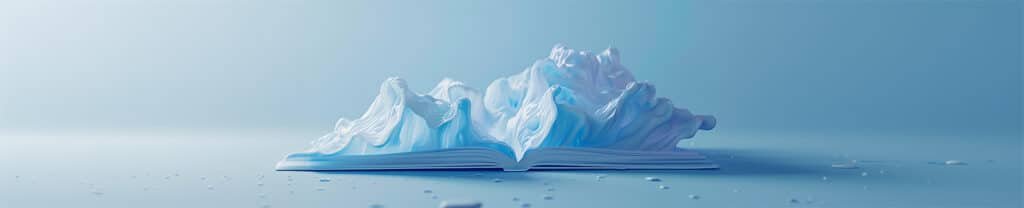
In 2024, artificial intelligence (AI) has become a must-have for companies looking to innovate, thanks in large part to the mass adoption of generative AI, capable of creating original content ranging from text to images to entirely new concepts. We’re not the first to say it: this technology opens up the prospect of an explosion […]
The importance of AI in business
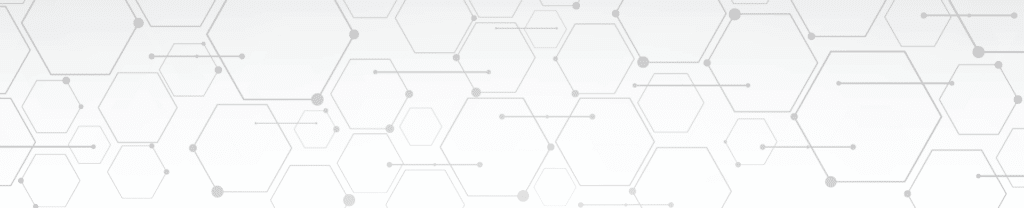
Just a few years ago, artificial intelligence was merely a playground reserved for innovative giants of Silicon Valley. However, the landscape has changed, and today, its evolution and democratization are undeniable: AI is the new industrial revolution. All companies must now include it in their operations to remain competitive over the next 5, 10, or […]
Generative Artificial Intelligence Applied in the Manufacturing Industry
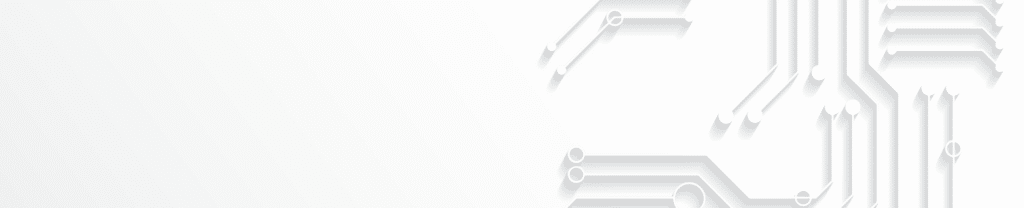
The Context The current challenges in the manufacturing industry in Quebec are numerous and complex. Disruptions in the supply chain, whether orchestrated by external global events such as the pandemic, trade conflicts, or internal issues like logistical dilemmas, can trigger disruptions, shortages of raw materials, and potentially result in financial losses equivalent to 45% of […]
Artificial Intelligence in Retail: The Essential List of Use Cases
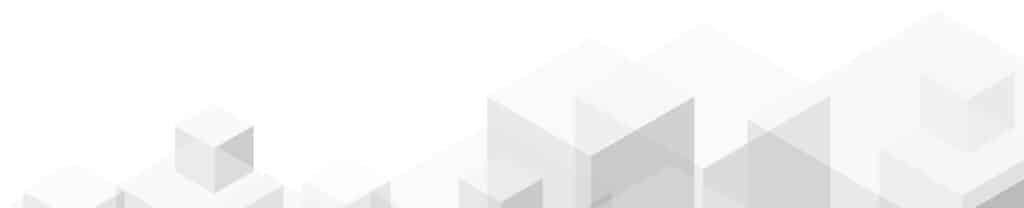
Anticipating future trends is the key to success for retail, food, and consumer goods (CPG) companies. And when it comes to prediction, artificial intelligence (AI) is emerging as the super fuel for this forecasting machine. Nothing is forever except change. Bouddha Managers dealing with seasonality, sudden changes in demand levels, events, supplier price fluctuations, strikes, […]
Automated Generation of Metadata: Solutions for SAP, Oracle, and Microsoft Dynamics Inventories

Imagine a scenario where the descriptions and categories of your inventory products are generated automatically. This prospect is now within reach thanks to generative artificial intelligence. Like any innovation project, the aim is to achieve increased productivity, reduced costs, better resource management, all with the ultimate goal of gaining a competitive advantage. And this is […]
The Essential Use Cases of Generative Artificial Intelligence

Generative artificial intelligence: why is everyone talking about it? With the growing popularity of generative artificial intelligence technologies, such as PaLM 2 and ChatGPT, more and more companies are seeking ways to integrate AI into their daily operations. According to a McKinsey report, generative AI will have a significant impact on the economy by increasing […]
Why you should use ChatGPT in a business context
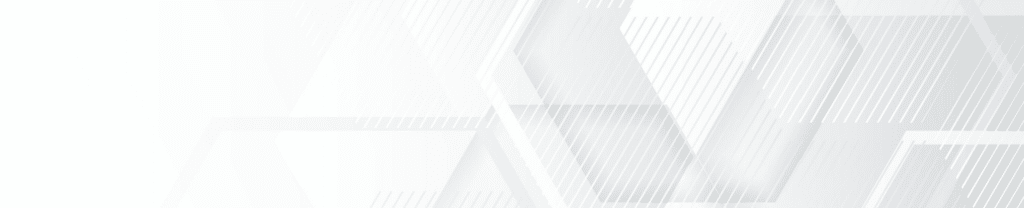
The challenges of integrating innovation like generative artificial intelligence solutions in your company OpenAI made a big impact in the field of artificial intelligence by unveiling ChatGPT, sparking a frenzy of adoption among millions of people. For the first time, we witnessed a true democratization of artificial intelligence. This innovation opened the eyes of everyday […]